Big Data Analytics: A Recent Break-Through In The E-Commerce Sector
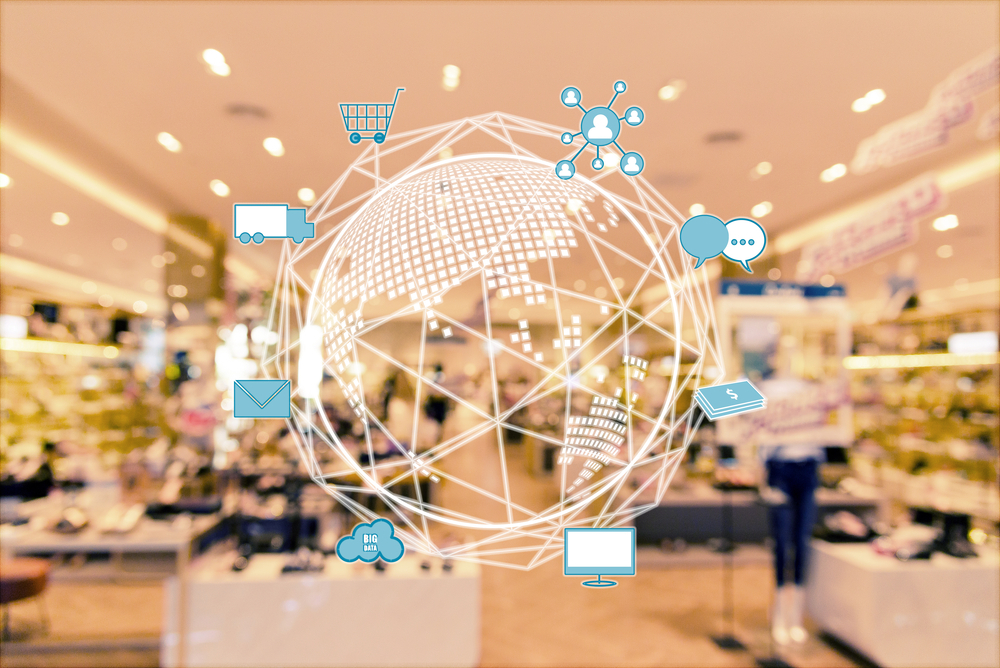
In the present digital age, an enormous amount of data is being rapidly generated and stored in computing systems at an incredible rate.
Consequently, to manage such an explosive variety of data, businesses are using Big Data Analytics to make profitable gains from the data produced.
BIG DATA ANALYTICS:
Big Data Analytics is literally the driving force behind everything we do online, applicable in every sector of the world.
Ever since the dawn of the internet, data has been growing exponentially, with more means to produce it (pictures, videos, audio, location, music, search history, shopping history, etc.) growing day by day. This is resulting in an overflow of information.
Organizations collect and store this information called Big Data, and then extract useful content from it which helps them in improving their services by providing valuable insights, predicting trends, and customer preferences.
To understand how Big Data Analytics works, and how it is transforming the e-commerce sector, we need to start with the basics.
What is Big Data?
Big Data refers to massive, diverse sets of information from various sources. It is used to describe large, complex, and hard-to-manage volumes of data that cannot be processed or analyzed by traditional data processing tools.
Big data is one of the top-growing sectors of the world. This technology collects and analyzes large quantities of data to produce actionable plans for companies to enhance their performances. It gives businesses a distinctive edge and has numerous benefits.
This is why companies, organizations, health sectors, governments, and businesses focus on Big data to increase their drive and growth.
Quick facts about Big Data:
- There are about 66 billion active internet users in the world.
- More than 5 quintillion bytes of data is generated every day.
- By 2025 463 ZB of data will be created every day.
- Data exists in numerous formats as it is coming from different sources like social media, news channels, banks, government, educational institutes, and many other industries.
- This extraordinary collection of data is called ‘BIG DATA’.
What are the important characteristics of Big Data?
Industries analyze Big data to generate meaningful insights, which helps them in strategizing and planning their next business move.
To comprehend how this technology works and where it can be utilized, it is vital to know Big data’s core characteristics. There are primarily five characteristics of Big Data Analytics:
Volume:
The first and the most commonly cited characteristic of big data is Volume. It is simply the amount of data present, commonly measured in Bytes (Gigabytes GBs, Zettabytes ZBs, and Yottabytes YBs). Organizations extract large amounts of data from various sources like clickstreams, bank sectors, smart devices, cell phones, industrial equipment, and more.
Previously, storing, processing, and managing all that data would have been too costly. But now platforms like Hadoop and the cloud gather and organize data efficiently.
Value:
Value is simply deriving meaningful and useful data from the huge sea of information, in other words, it is the benefit that the data adds to your organization.
Velocity:
Velocity simply describes the speed at which data is generated and processed. It comprises the rate of change, activity bursts, and connections between incoming data sets that are to be handled in a timely manner. High velocity is essential for the effective execution of any Big Data process.
Variety:
Variety is defined as various formats of data coming in from diverse sources. It is necessary to manage the variety of your and store it in an organized way. Unorganized data severely affects performance and is one of the most common issues of data processing.
Veracity:
Lastly, veracity is how accurate and true your data is. It communicates the level of reliability of the data available. Veracity is the most important characteristic of Big data, as low veracity of data can lead to false and inaccurate results.
What are the types of Big Data?
The data stored in the Big Data reservoirs can be categorized into 6 types.
Structured data:
Data that is organized, well-defined, follows a consistent order, has a clear structure, and conforms to a data standard is called structured data.
This type of data is the easiest to manage and can be easily accessed by an organization, computer, or person.
A common example of structured data is the information businesses keep in their datasets in the form of spreadsheets and tables.
Unstructured data:
The majority of big data comes falls into this category, it is defined as the type of data that is heterogenous, unorganized, and doesn’t follow a data model.
Unstructured data is the complete opposite of structured data, this data cannot be easily accessed by a system or person and is extremely chaotic and hard to manage.
Social media posts, comments, snaps, snaps stories, tweets, YouTube views, and WhatsApp messages are examples of Unstructured Big Data.
Semi-structured data:
Semi-structured, as the name suggests, is the combination of both structured and unstructured data. This data doesn’t follow a data model but has some structure.
Semi-structured data cannot be stored in rows and columns like structured data but has some organizational elements that make it much less chaotic to handle than unstructured data.
Emails, Zipped files, Web pages, Binary executables, XML, and other markup languages are some sources of semi-structured data
Geospatial data:
Geospatial or spatial data is the information that represents the events, features, phenomena, or objects located on the earth’s surface.
It is basically used to represent any type of data that has a geographic component, this information can be about the weather, earthquakes, addresses, streets, traffic, accidental data, places of interest, epidemics, or pandemics.
Image credits: Google Data Analytics
Machine data:
Machine or operational data is the data that is produced by machines without human contribution. This type of data is growing at an incredible rate and has a significant impact on all industries that use computers in their daily operations.
Machine data is an important type of data as machines generate more data than people do. Atm transactions, sensors, calculations, predictions, automation, and metadata are some types of machine data.
Open-source data:
Open-source data are datasets that can be openly accessed, edited, and shared by the public for any purpose. This type of data is readily available to anyone with an internet connection.
Open-source databases are open to sharing and free, the users of open-source databases can construct systems according to their own needs and preferences.
Image credits: US Census Bureau
What is meant by Big Data Analytics (BDA)?
Big Data Analytics is referred to examining large and different sets of data to uncover hidden patterns, correlations, and other insights.
Big Data Analytics involves tools that not only help organizations manage their data with efficiency but also significantly contribute to leveraging their performances.
In simple words, Big data analytics is collectively helping large corporations to facilitate their growth and development. This involves applying data mining and machine learning algorithms which result in better decisions, innovations, and increased sales.
What are the Big Data Technologies? And how do they Work?
Big Data technologies are the sources, software and tools that are used to manage all types of datasets and transform them into business insights, they can be split into 5 Categories:
Big Data Sources:
All successful corporations depend on various sources and find new ways to extract and collect data. These datasets are from prominently two types of sources.
- Internal data sources: Data from these sources attained from the internal reports from an organization. Some good examples of internal sources of data are annual sales and profit reports of an organization.
- External data sources: Data that comes from outside an organization’s database is called external data. Examples include government statistics, regional data, new reports, etc.
Big Data Storage:
Big data storage technologies are tools that are designed to store, manage and access large quantities of data smoothly. Different applications are used to store data some of which are:
Big Data Mining:
Big data mining refers to the process of extracting useful information from a huge set of raw data. Data mining software enables us to extract valuable information using techniques such as statistical analysis, machine learning, predictive analysis, etc.
The main goal is to find insight and predict trends that will result in more effective marketing and sales.
The top big data mining technologies are:
Big Data Analytics:
These software are specifically designed with powerful analytical tools, they examine and clean data so that the companies can answer business queries and improve results.
Big data analysis software discovers correlations, and hidden patterns and provides insights to users in a timely manner.
Some of the best Big data analytics tools are:
Data Visualization:
Another type of data analytics technology that is developed specifically for analyzing data and presenting it in visually appealing manners like graphs, charts, diagrams, maps, or infographics.
Data visualization software are:
Some other prevalent and effective Big Data analytics tools and visualization software are:
- Zoho Analytics
- Yellowfin
- Qlik Sense
- Reveal
- Domo
- GoodData
- MATLAB
- IBM Watson
- Datawrapper
- Minitab
- Splunk
- Orange
- Elasticsearch
- Qubole
- Lumify
- Stata
- Birst
- Microsoft Power BI
- BigML
- Statwing
- Plotly
What are the stages of Big Data Analysis?
After knowing what is big data and Big data analytics, let us have a look at what are the stages involved in this impressive process:
Identifying the problem:
The first and the most important step is obviously to identify the issue. It involves asking important questions like what exactly is our problem and can it be addressed.
Designing data Requirement:
After identifying the problem, the second step is to decide what kind of data is required to solve this particular matter.
Pre-processing Data:
In this step data is cleaned and processed a bit.
Performing Analytics over Data:
Fourth step involves data analysis using various tools and methods.
Visualizing Data
In the final stage the analyzed data is presented in a proper visual format.
The Types of Big Data Analytics:
Big data analytics is divided into 4 main types:
- Descriptive Analytics
- Diagnostic Analytics
- Predictive Analytics
- Prescriptive Analytics
How is Big Data Analysis Helping Organizations all around the globe?
Smarter and more Intelligent Organizations:
Big data analytics is significantly contributing to making organizations smarter and more efficient, and organizations are adopting this technology to lead them into making faster decision making. For example:
New York City Police Department (NYPD) uses Big data Analytics to prevent criminal activities in advance.
They identify patterns, criminal histories, geolocations to reveal crime hotspots in the city, anticipate crimes, deploy their officers on the locations and catch the criminals even before the crime is committed.
Image credits: New York City Comptroller
Optimizing e-commerce operations by Analyzing Customer behavior:
Big Data Analysis (BDA) enhances Businesses by monitoring and analyzing customer behavior and preferences, industries utilize this data for improving their customer service and expanding their businesses. Best example of this is Amazon.
They do so by:
- Click stream Analysis: Analyzing the clicks of every visitor on the website.
- Studying Paths leading to purchases: they study the paths that lead customers to buy products and also what makes them leave.
Image credits: Amazon Warehouse
Cost Reduction:
Storage software like Hadoop technologies reduce the price for storing data by eliminating the need for hardware devices.
For example, Parkland Land hospital in Dallas Texas, uses big data storage tools to store patient information in the form of Electronic health records (ERC). It also uses data analytics and predictive modeling to identify high risk patients and contribute in prevent diseases.
Understanding Customer preferences:
Starbucks caters to the demands of their customers and satisfies them by studying the purchasing habits using Big data.
Next-Generation Products:
Big Data analytic technologies help in development of high-tech next generation products and services like Google Self-driving cars, and Netflix.
Future Foresight:
In the near future, Business Analytic tools and Artificial intelligence techniques will be seen everywhere, and will be applicable in every facet of our lives.
Major sectors of the world including banking, finance, e-commerce, healthcare and governments would be highly dependent on BDA software.
Hence in the upcoming years applying Big data Analytic tools will be an inevitable necessity for businesses and individuals to extract valuable information and generate insights.
We are about to witness a fundamental revolution in our lifestyle and businesses due to this epic technology.
